Overview | Organizers | Invited Speakers | Conference Program | Book of Abstracts | Useful Information | Social Program
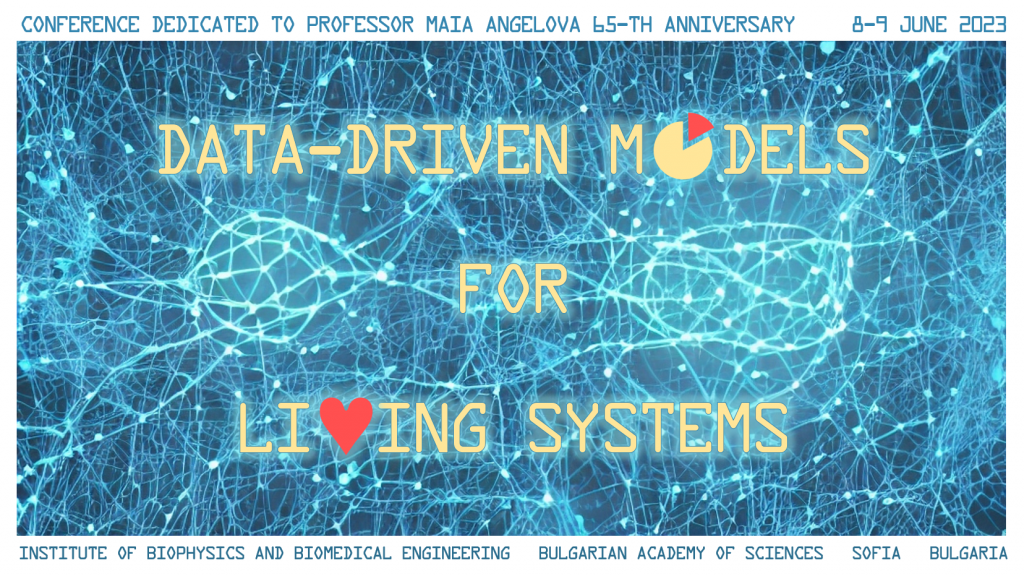
Invited Speakers
Professor Maia Angelova, Data Analytics Research Lab, School of Information Technology, Deakin University, Melbourne Burwood Campus, Australia
“Data-Driven Models for Precision Healthcare”
Abstract
Precision healthcare is an emerging approach for personal healthcare and disease prevention that considers the individual’s variability in health, genes, lifestyle, and environment. For example, sleep and nutrition are essential and repeating processes which are vital for the quality of life and well-being of every individual. These processes involve complex dynamics, interactions between organs and environment, and regulation at multiscale that reflect the developmental changes in mental and physical health, along with the day-to-day fluctuations. In this talk, I will consider the potential of hybrid data-driven models and artificial intelligence in multiscale modelling for patient-centered digital healthcare of the future.
Professor Alejandro Frank, Center for Complexity Science and Nuclear Science Institute, UNAM, Mexico (online)
“Symmetry and Criticality in Biological Systems: a New Approach to Multisignal Analysis”
Abstract
In biology, criticality refers to the phenomenon where a system or network of components is poised at a point between order and disorder, with the potential for rapid transitions or changes in behavior. This critical state is thought to be a key feature of many biological systems, from the functioning of the brain to the behavior of ecosystems. In this talk I present a multisignal time series analysis based on scale invariance of the correlation matrices and discuss some applications.
Professor Anatoli Ivanov, Pennsylvania State University, USA
“Differential Delay Models in Biology”
Abstract
Differential delay equations are frequently used as mathematical models of diverse real-world phenomena where the time delays are intrinsic features of their functioning. In the past half a century they were particularly useful in adequate modeling of various biological processes, especially in population dynamics and physiology.
Two particular differential delay equations of interest are a model of hematopoiesis (blood cell production) [4] and a model of megakaryopoiesis (platelet production) [1]. Both models are described mathematically by simple form scalar differential delay equations, which are closely related by several properties. Dynamics of solutions in these equations are comprehensively studied. Sufficient conditions are derived when the unique positive equilibrium is globally asymptotically stable [2]. The stability conditions are given in terms of induced interval maps, one set being delay independent criteria and another one involving the size of delay.
The existence of periodic solutions slowly oscillating about the equilibrium is also established [3]. The periodic oscillations in the models always exist when the positive equilibrium is linearly unstable. The proof of existence follows the established ejective fixed point techniques with necessary modifications due to the specific form of the equations.
References
[1] L. Boullu, M. Adimy, F. Crauste, and L. Pujo-Menjouet, Oscillation and asymptotic convergence for a delay differential equation modeling platelet production, Discrete and Continuous Dynamical Systems, Ser. B, 24:6, 2417-2442, 2019.
[2] A. Ivanov, Global asymptotic stability in a differential delay equation modeling megakaryopoiesis, Functional Differential Equations, 28:3-4, 103-116, 2021.
[3] A. Ivanov and B. Lani-Wayda, Periodic solutions in a differential delay equation modelling megakaryopoiesis, Proceedings of ISAAC-2021, Springer-Verlag, 12 pp. (to appear)
[4] M.C. Mackey, Unified hypothesis of the origin of aplastic anaemia and periodic hematopoiesis, Blood, 51: 941-956, 1978.
Professor Aneta Stefanovska, Lancaster University, UK
“Can Non-autonomous Dynamics in Mathematics Adequately Describe Open Systems in Physics? Lessons from Data Analysis in Cellular, Cardiovascular and Brain Dynamics”
Abstract
Physics distinguishes between isolated systems (no exchange of energy or matter with the environment), and non-isolated systems. The latter can either be closed, only able to exchange only energy with the environment, or open in which case they can exchange both energy and matter with the environment. Already by 1943 during his lectures delivered under the auspices of the Dublin Institute for Advanced Studies where he was Director of Theoretical Physics, at Trinity College, Dublin, Erwin Schrödinger pointed out that
…living matter, while not eluding the “laws of physics” as established up to date, is likely to involve “other laws of physics” hitherto unknown, which however, once they have been revealed, will form just as integral a part of science as the former.
Schrödinger furthermore clarified [1] that living matter evades the decay to thermodynamic equilibrium by homeostatically maintaining negative entropy in an open system.
However, even today, we do not have the new laws of physics needed to describe living systems. Open systems are usually treated within the framework of statistical physics and stochastic dynamics, and the data measured from such systems are increasingly analysed using artificial intelligence.
In this talk we will propose oscillatory non-autonomous dynamical systems ([2] as potential candidates to describe open systems. The talk will consists of four parts. First, we will consider several examples on a variety of timescales and size scales – including cellular systems up to the cardiovascular and brain dynamics, as well as diurnal oscillations and population levels [3-6], to illustrate the motivation for our proposal. Secondly, we will introduce chronotaxic (chronos – time, taxis – order) systems [7], proposed to provide a framework for non-autonomous systems that can endure continuous external perturbation, focusing on the properties of frequency-modulated dynamical systems and networks. We will illustrate that oscillators subject to driving with a slowly varying frequency, counterintuitively, have enlarged Arnold tongue in the parameter space [8]. Thirdly, we will present an experimental model of electrons on the surface of liquid helium subject to an electromagnetic field and microwaves that exhibit chronotaxic dynamics [9]. So far, chronotaxic dynamics has been observed mainly in living systems allowing only very limited possibilities for studying experimentally the effect of changing relevant parameter values. In contrast to these biological observational studies, the superfluid helium experiments offer us an opportunity to gain an understanding of chronotaxic behavior based on well-controlled laboratory investigations that promise to reveal fully the relationship between the physical world and the fundamental nature of chronotaxic dynamics. Lastly, we will introduce the idea of describing living systems as networks of networks of chronotaxic systems. We will propose that the conservation of phase difference could be the new law of physics that underlines living systems.
References
[1] Erwin Schrödinger (1944), What Is Life? and Other Scientific Essays. Based on lectures delivered under the auspices of the Dublin Institute for Advanced Studies at Trinity College, Dublin, in February 1943. Doubleday (1956) and Internet Archive.
[2] Peter Kloeden and Martin Rasmussen (2011) Nonautonomous Dynamical Systems, American Mathematical Society, Providence.
[3] Maja Bračič Lotrič and Aneta Stefanovska (2000) Synchronization and modulation in the human cardiorespiratory system, Physica A 283, 451.
[4] Francisco Varela et al (2001) The brainweb: Phase synchronization and large-scale integration, Nature Reviews Neuroscience 2, 229–239.
[5] Gemma Lancaster et al (2016) Modelling chronotaxicity of cellular energy metabolism to facilitate the identification of altered metabolic states, Scientific Reports 6 (1), 1-12.
[6] Megan Morris, Shin Yamazaki and Aneta Stefanovska (2022) Multiscale time-resolved analysis reveals remaining behavioral rhythms in mice without canonical circadian clocks, Journal of Biological Rhythms 37 (3), 310-328.
[7] Yevhen Suprunenko, Philip Clemson and Aneta Stefanovska (2013) Chronotaxic systems: A new class of self-sustained nonautonomous oscillators, Physical Review Letters 111 (2), 024101.
[8] Maxime Lucas, Julian Newman, Aneta Stefanovska (2018) Stabilization of dynamics of oscillatory systems by nonautonomous perturbation, Physical Review E 97 (4), 042209.
[9] Hala Siddiq et al (2023) Visualization of oscillatory electron dynamics on the surface of liquid helium, Physical Review B 107, 104501.
Professor Cristopher Stephens, UNAM, Mexico (online)
“The Conductome – A New Paradigm for Understanding Human Behaviour”
Abstract
As almost every major problem that humankind faces are a consequence of human behaviour, predicting behaviour and behaviour change is fundamental. Given the multitude of factors that affect our decision making, a transdisciplinary understanding of behaviour is impossible without the integration of data that cross disciplinary boundaries. The concept of Conduct-“ome” is an analog of those holistic –“omic”-approaches found in the biological sciences which take a “totality of factors” approach, and provides a framework for studying human behaviour in a multifactorial, multidisciplinary context, accounting for a wealth of potential causes of behaviour, from the genetic and epigenetic to psychological, neurological, social, physiological, clinical, socio-economic, socio-demographic, socio-political and ethical factors. Conductome, as opposed to behaviour-ome, is used, as it directly addresses the “whys” (causes) of the considered behaviour. We argue that behaviour can only be understood probabilistically, through a process of statistical inference that constructs P(A|X), the probability for a conduct A conditioned on the large set of factors, X, that predict it. This inference process can be based on an “external” ensemble of objective, countable events, using a frequentist interpretation of probabilities, or on an “internal” ensemble, implicit in our mental models and based on a Bayesian interpretation. Including both these approaches allows one to compare objective, observable reality with the subjective perception of reality constructed within a mental model, allowing for the identification of discrepancies between the two in the form of cognitive biases. A key component for constructing the Conductome is the obtention of data that transcends disciplines, and which can be used to link a range of relevant behaviours, as effects, to their causes, both internal and external. A second component is the use of advanced modelling tools, such as machine learning, for the analysis of such multi-scale data and the construction of explicit prediction models for a given conduct. In this article, the feasibility of the Conductome approach is illustrated by considering obesity-related behaviours; as obesity has become one of the key social problems that affects a growing segment of the population worldwide. In summary: The objective is to understand, interpret and provide an interdisciplinary, computational and data-based framework for generating prediction models for addressing problems that originate in human behaviour.
Professor Gang Li, Deakin University, Australia
“Bridging Topological Data Analysis and Machine Learning”
Abstract
Clustering is a powerful technique for discovering patterns and hidden structures in data. However, many real-world datasets contain clusters with varied densities and irregular shapes, which pose challenges for traditional clustering algorithms. Kernel-based clustering is a technique designed to identify non-linearly separable clusters with irregular shapes, but they have high computational costs. In this talk, I will present some recent advances in kernel-based clustering that use an adaptive distributional kernel to overcome the limitations of existing clustering algorithms. These methods can achieve better clustering quality with lower computational cost than existing kernel-based algorithms and can handle large datasets efficiently. Their applications can range from image and speech processing to gene pattern extraction, community detection and market segmentation.
Professor Gleb Beliakov, Deakin University, Australia (online)
“Random Generation of Fuzzy Measures for Simulations in Decision Making”
Gleb Beliakov, Simon James, Jian-Zhang Wu
Abstract
Non-additivity of fuzzy measures offers a formal mathematical treatment of interdependencies of various inputs. In the context of multiple criteria decision making they model redundancies and synergies among the decision criteria. The weights are assigned to the individual criteria and all their possible subsets, at the cost of exponential number of parameters. Even with some simplification strategies the number of importance weights are often too high. Simulation studies allow one to select the most frequent decision outcomes based on a large number of suitable fuzzy measures. For this we need a reliable technique of random generation of fuzzy measures, which translates into uniform generation of random points in high-dimensional polytope. This talk presents various approaches to that challenging problem.
Professor Kai Ming Ting, Nanjing University, China (online)
“Distributional Kernels: An Under-Utilized Resource”
Abstract
This seminar presents recent works on distributional kernels based on kernel mean embedding (KME). KME has a strong theoretical underpinning, and guarantees that the resultant kernel mean map is injective, i.e., the kernel mean maps of two distributions have their difference equals to zero if and only if the distributions are the same. Yet, KME’s applications have been less successfully so far. One key breakthrough is the identification of the root cause of KME’s (seemingly) failures, i.e., the use of Gaussian kernel. The seminar presents works, following this identification, that release the power of this under-utilized resource. The works demonstrate that the distributional kernels can solve long-standing problems, some of which have evaded decades of effort, in terms of efficiency and task-specific accuracy issues. These include point and group anomaly detections, clustering, and anomaly detections in trajectories, periodic time series and graphs/networks.
Professor Krassimir Atanassov, IBPhBME-BAS, Bulgaria
“On Self-Organization of Generalized Nets”
Abstract
Generalized Nets (GNs) are the most powerful extension of the Petri nets and the rest of their extensions and modifications. The GN tokens enter the net with initial characteristics and obtain next ones during the time of their transfer through the net, collecting their own history. Moreover, the GN transitions can have special matrices with predicates that determine whether a token from some input place can transfer to an output place or not. Following R. Valk’s idea for self-modifying Petri nets that can change their structure (at some moments some arcs of the net can disappear, at others – they can appear), in the end of the 1980s, self-modifying GNs were constructed. They had the capability to change both their structure and their behaviour (change affecting the functions that give next token characteristics, the predicates associated with the GN transitions, and others). These modifications of the GN-structure and self-organization of its behaviour are based on the operators, defined over GNs.
In the present paper, we discuss another approach to the implementation of self-modification (in Valk’s sense) and self-organization of the behaviour of a given GN. It is based only on the possibilities of the transition predicates and of the tokens characteristic functions to have as arguments values of other token characteristics and situations, that have occurred in the net by the present time-moment. This GN has three contours: first one – that represents a concrete real process; second one, that estimates the values of the token characteristics of all or at least a part of the tokens from the first contour and of the evaluations of the transition predicates obtained by the current time-moment; and third one – that determines possibilities for the change of some characteristic functions or transition predicates with the aim of correcting the current parameters of the first contour. In this third contour, a decision for stopping of the process in the first contour can be generated, if some parameters of the first contour are irreversibly changed.
Professor Peter McClintock, Lancaster University, UK
“Coulomb Blockade and Selectivity in Biological Ion Channels”
Abstract
Biological ion channels are essential to life in all its forms. By enabling the selective transfer of ions across lipid bilayers, they facilitate the establishment of intracellular media able to support the biochemical reactions involved in metabolism and replication. Their selectivity for particular kinds of ions is known to be associated with a fixed negative charge
Qf in a narrow part of the channel known as the selectivity filter. Remarkably, many properties of ion channels can now be understood [1] by analogy with the physics of quantum dots: e.g. Coulomb blockade oscillations in quantum dots correspond to the alternating conduction bands and stop bands seen for ions in channels as
Qf is varied – emergent phenomena that could not have been anticipated based on the known channel structure. The Coulomb blockade model will be summarised and some systematic experimental and numerical tests [2] will be discussed, together with more recent work developing a fundamental statistical theory [3] of the conduction process.
References
[1] I. Kh. Kaufman, P. V. E. McClintock, and R. S. Eisenberg, New J. Phys. 17 (2015) 083021.
[2] O. A. Fedorenko, I. Kh. Kaufman, W. A. T. Gibby, M. L. Barabash, D. G. Luchinsky, S. K. Roberts, and P. V. E. McClintock, Biochim. Biophys. Acta 1862 (2020) 183301.
[3] W. A. T. Gibby, M. L. Barabash, C. Guardiani, D. G. Luchinsky, and P.V.E. McClintock, Phys. Rev. Lett. 126 (2021) 218102.
Professor Reidun Twarock, University of York, UK (online)
“A Tale of Symmetry: From Viruses to Protein Nanoparticles”
Abstract
Viruses are examples of symmetry in biology. The majority of viruses package, and thus protect, their gnomes in protein cages, called capsids, that are organised according to icosahedral symmetry. Protein nanoparticles, either derived from viruses or de novo designed, play pivotal roles in a host of applications, including drug delivery, vaccination and diagnostics. Mathematical Virology has provided unprecedented insights into the structure, self-assembly and evolution of viral capsids. Exploiting synergies between virology and protein nanotechnology, I will demonstrate how this work informs the rational design of protein nanoparticles for diverse applications.
Dr Chandan Karmakar, Deakin University, Australia
“Impact of Knowledge-Based Filtering on Clinical Decision-Making System — Arrhythmia Detection Case Study”
Abstract
The Electrocardiogram (ECG) is a vital signal used to monitor heart conditions, but it is prone to contamination by various types of noise. Traditional approaches for filtering ECG noise rely on predefined filters, which often lead to the unintentional removal of noise-free signals. To overcome this challenge, we present a novel framework that automatically detects and classifies ECG signals based on the presence and types of noise, enabling the application of tailored filtering techniques.
We demonstrate the advantages of employing noise-aware and noise-type-based filtering approaches compared to noise-unaware filtering by quantifying the distortions in ECG biomarkers, specifically the QT and QRS intervals, caused by the filtering process. Our experimental results showcase the effectiveness of our hierarchical model, utilizing Adaboost, in achieving exceptional performance. The model achieved a 100% accuracy rate for noise detection and an impressive 97.19% accuracy rate for noise classification. These results further validate the efficacy of our approach in accurately identifying and categorizing different types of noise present in ECG signals.
The reported results were obtained using the inter-dataset splitting approach, employing six diverse datasets called CINC-2011, CINC-2014, ECG-ID, MIT-Bih arrhythmia, BIDMC, and teleECG. Our investigation focused on comparing the performance of noise-aware, noise-unaware, and noise-type-based filtering techniques. Notably, the noise-type-based filtering approach exhibited the smallest mean difference (2.50ms) in the QT interval, surpassing both noise-aware (−16.37ms) and noise-unaware (−38.23ms) filtering methods. Moreover, for the QRS interval, noise-unaware filtering produced the highest mean difference (−10.33ms), whereas this was significantly reduced to −2.43ms and 4.28ms with noise-aware and noise-type-based filtering, respectively. These outcomes convincingly demonstrate the superiority of noise-aware and noise-type-based filtering approaches in preserving the integrity of clinical biomarkers in ECG signals.
We also used our knowledge-based filter framework for arrhythmia detection and compare the result with noise-unaware and noise-aware filter to show the significance of knowledge-based filtering system.
Dr Rubén Fossión, UNAM, Mexico
“Frailty Syndrome as a Transition from Compensation to Decompensation: Application to the Biomechanical Regulation of Gait”
Abstract
Most gait parameters decrease with age and are even more importantly reduced with frailty. However, other gait parameters exhibit different or even opposite trends for aging and frailty, and the underlying reason is unclear. Literature focuses either on aging, or on frailty, and a comprehensive understanding of how biomechanical gait regulation evolves with aging and with frailty seems to be lacking. We monitored gait dynamics in young adults (19–29 years, n = 27, 59% women), middle-aged adults (30–59 years, n = 16, 62% women), and non-frail (>60 years, n = 15, 33% women) and frail older adults (>60 years, n = 31, 71% women) during a 160 m walking test using the triaxial accelerometer of the Zephyr Bioharness 3.0 device. Frailty was evaluated using the Frail Scale (FS) and the Clinical Frailty Scale (CFS). We found that in non-frail older adults, certain gait parameters, such as cadence, were increased, whereas other parameters, such as step length, were decreased, and gait speed is maintained. Conversely, in frail older adults, all gait parameters, including gait speed, were decreased. Our interpretation is that non-frail older adults compensate for a decreased step length with an increased cadence to maintain a functional gait speed, whereas frail older adults decompensate and consequently walk with a characteristic decreased gait speed. We quantified compensation and decompensation on a continuous scale using ratios of the compensated parameter with respect to the corresponding compensating parameter. Compensation and decompensation are general medical concepts that can be applied and quantified for many, if not all, biomechanical and physiological regulatory mechanisms of the human body. This may allow for a new research strategy to quantify both aging and frailty in a systemic and dynamic way.
Dr Sergiy Shelyag, Flinders University, Australia
“Decision-Making in Complex Competitive Environments: From Wars to Soccer”
Sergiy Shelyag, Maia Angelova, Ishara Bandara, Abhiram Thiruthummal, Mathew Zuparic, Alex Kalloniatis, Dan Dwyer, Shitanshu Kusmakar, Chandan Karmakar, Sutharshan Rajasegarar, Ye Zhu
Abstract
How do people make and implement decisions? Decisions are based on time-dependent interactions with the environment, which is invariably complex, volatile, and uncertain. This is applicable to any kind of decision-making, including competitive sports, co-operational and competitive organisational structures, military conflicts. Characterising and modelling decision-making processes and designing model-informed strategies for organisational structures with the aim to achieve favourable results, therefore, would be of interest. In my presentation, I will show a mathematical model of decision-making, which is based on interactions between agents of different kinds. The interactions are modelled to be adversarial, supportive and contributing. The model is studied both analytically and numerically, and I will discuss applicability of the model to a variety of real-life scenarios.
Dr Ye Zhu, Deakin University, Australia
“Discovering Meaningful Clusters from Massive Datasets Using Distributional Kernel”
Abstract
Topological data analysis (TDA) extracts the topological features that are complementary to statistical quantities, which has been found in many applications in computer vision. In TDA, Persistence diagram (PD) has been considered as a compact descriptor for topological data analysis (TDA). Unfortunately, PD cannot be directly used in machine learning methods since it is a multiset of points. Recent efforts have been devoted to transforming PDs into vectors to accommodate machine learning methods. However, existing methods share one common shortcoming: the mapping of PDs to a feature representation depends on a pre-defined polynomial. This presentation will introduce two recent advances: polynomial representation and Hilbert representation for PD, with the aim of extracting the discriminative topological features. Finally, potential applications in the field of computer vision, and biomedical science will be discussed.